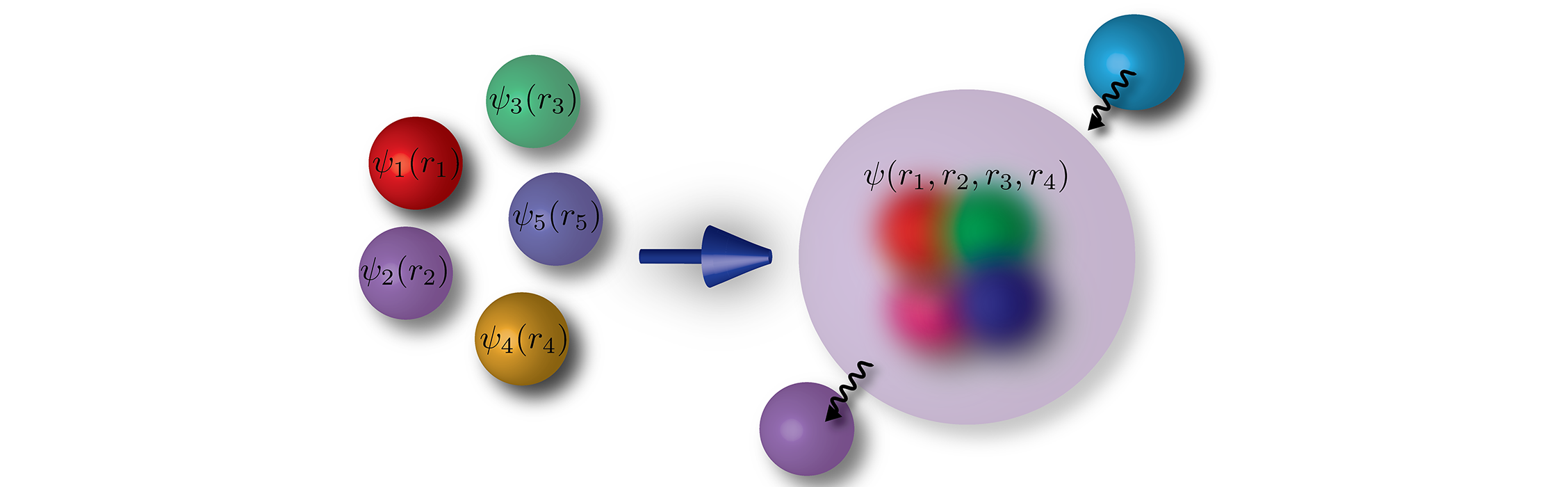
Our group aims to theoretically AND experimentally investigate various quantum properties of light-matter interaction for applications in future optoelectronic devices, quantum information processing, and sensing. Moreover, we explore associated fundamental phenomena, such as many-body physics, that could emerge in such physical systems. Our research is at the interface of quantum optics, condensed matter physics, quantum information sciences, and more recently, machine learning.
Jingchen Zhang
Research Areas:
- Quantum Information Science
- Hybrid quantum systems
Peter Lunts
Research Areas:
- Quantum Information Science
- Many-body physics
- Hybrid quantum systems
Supratik Sarkar
I am a PhD student at Joint Quantum Institute (JQI), Institute for Research in Electronics and Applied Physics (IREAP), University of Maryland working under the supervision of Dr. Mohammad Hafezi.
Hafezi Elected APS Fellow
JQI Fellow Mohammad Hafezi has been elected as a Fellow of the American Physical Society (APS). He was cited for “pioneering theoretical and experimental work in topological photonics and quantum synthetic matter.”
Novel Design May Boost Efficiency of On-Chip Frequency Combs
On the cover of the Pink Floyd album Dark Side of the Moon, a prism splits a ray of light into all the colors of the rainbow. This multicolored medley, which owes its emergence to the fact that light travels as a wave, is almost always hiding in plain sight; a prism simply reveals that it was there.
Compressed Sensing Measurement of Long-Range Correlated Noise (Short Talk + Pre-Print)
Long-range correlated errors can severely impact the performance of NISQ (noisy intermediate-scale quantum) devices, and fault-tolerant quantum computation. Characterizing these errors is important for improving the performance of these devices, via calibration and error correction, and to ensure correct interpretation of the results.
JQI Researchers Generate Tunable Twin Particles of Light
Identical twins might seem “indistinguishable,” but in the quantum world the word takes on a new level of meaning. While identical twins share many traits, the universe treats two indistinguishable quantum particles as intrinsically interchangeable. This opens the door for indistinguishable particles to interact in unique ways—such as in quantum interference—that are needed for quantum computers. While generating a crowd of photons—particles of light—is as easy as flipping a light switch, it’s trickier to make a pair of indistinguishable photons. And it takes yet more work to endow that pair with a quantum mechanical link known as entanglement. JQI researchers and their colleagues describe a new way to make entangled twin particles of light and to tune their properties using a method conveniently housed on a chip, a potential boon for quantum technologies that require a reliable source of well-tailored photon pairs.
Machine learning the thermodynamic arrow of time published in Nature Physics
The mechanism by which thermodynamics sets the direction of time’s arrow has long fascinated scientists. Here, we show that a machine learning algorithm can learn to discern the direction of time’s arrow when provided with a system’s microscopic trajectory as input. The performance of our algorithm matches fundamental bounds predicted by nonequilibrium statistical mechanics.